203: Exampville Destination Choice
Contents
203: Exampville Destination Choice¶
Welcome to Exampville, the best simulated town in this here part of the internet!
Exampville is a demonstration provided with Larch that walks through some of the data and tools that a transportation planner might use when building a travel model.
import larch.numba as lx
from larch import P, X
In this example notebook, we will walk through the estimation of a tour destination choice model. First, let’s load the data files from our example.
hh, pp, tour, skims, emp = lx.example(200, ['hh', 'pp', 'tour', 'skims', 'emp'])
For this destination choice model, we’ll want to use the mode choice
logsums we calculated previously from the mode choice estimation,
but we’ll use these values as fixed input data instead of a modeled value.
We can load these logsums from the file in which they were saved.
For this example, we can indentify that file using the larch.example
function, which will automatically rebuild the file if it doesn’t exists.
In typical applications, a user would generally just give the filename
as a string and ensure manually that the file exists before loading it.
logsums_file = lx.example(202, output_file='logsums.zarr.zip')
logsums = lx.DataArray.from_zarr('logsums.zarr.zip')
Preprocessing¶
The alternatives in
the destinations model are much more regular than in the mode choice
model, as every observation will have a similar set of alternatives
and the utility function for each of those alternatives will share a
common functional form. We’ll leverage this by using idca
format
arrays in our DataTree to make data management simpler.
The base array we’ll start with will have two dimensions, cases and alternatives, anc be formed from the logsums we loaded above.
ca = lx.Dataset.construct(
{'logsum': logsums},
caseid='TOURID',
alts=skims.TAZ_ID,
)
ca
<xarray.Dataset> Dimensions: (TAZ_ID: 40, TOURID: 7564) Coordinates: * TAZ_ID (TAZ_ID) int64 1 2 3 4 5 6 7 8 9 10 ... 32 33 34 35 36 37 38 39 40 * TOURID (TOURID) int64 0 1 3 7 10 13 ... 20728 20729 20731 20734 20736 Data variables: logsum (TOURID, TAZ_ID) float64 dask.array<chunksize=(1891, 20), meta=np.ndarray> Attributes: _caseid_: TOURID _altid_: TAZ_ID
For our destination choice model, we’ll also want to use employment data. This data, as included in our example, has unique values only by alternative and not by caseid, so there are only 40 unique rows. (This kind of structure is common for destination choice models.)
emp.info()
<class 'pandas.core.frame.DataFrame'>
Int64Index: 40 entries, 1 to 40
Data columns (total 3 columns):
# Column Non-Null Count Dtype
--- ------ -------------- -----
0 NONRETAIL_EMP 40 non-null int64
1 RETAIL_EMP 40 non-null int64
2 TOTAL_EMP 40 non-null int64
dtypes: int64(3)
memory usage: 1.2 KB
Then we bundle all our raw data into a DataTree
structure,
which is used to collect the right data for estimation. The
Larch DataTree is a slightly augmented version of the regular
sharrow.DataTree
.
tree = lx.DataTree(
base=ca,
tour=tour.rename_axis(index='TOUR_ID'),
hh=hh.set_index("HHID"),
person=pp.set_index('PERSONID'),
emp=emp,
skims=lx.Dataset.construct.from_omx(skims),
relationships=(
"base.TAZ_ID @ emp.TAZ",
"base.TOURID -> tour.TOUR_ID",
"tour.HHID @ hh.HHID",
"tour.PERSONID @ person.PERSONID",
"hh.HOMETAZ @ skims.otaz",
"base.TAZ_ID @ skims.dtaz"
),
)
Model Definition¶
Now we can define our choice model, using data from the tree as appropriate.
m = lx.Model(datatree=tree)
m.title = "Exampville Work Tour Destination Choice v1"
m.quantity_ca = (
+ P.EmpRetail_HighInc * X('RETAIL_EMP * (INCOME>50000)')
+ P.EmpNonRetail_HighInc * X('NONRETAIL_EMP') * X("INCOME>50000")
+ P.EmpRetail_LowInc * X('RETAIL_EMP') * X("INCOME<=50000")
+ P.EmpNonRetail_LowInc * X('NONRETAIL_EMP') * X("INCOME<=50000")
)
m.quantity_scale = P.Theta
m.utility_ca = (
+ P.logsum * X.logsum
+ P.distance * X.AUTO_DIST
)
m.choice_co_code = "tour.DTAZ"
m.lock_values(
EmpRetail_HighInc=0,
EmpRetail_LowInc=0,
)
Model Estimation¶
m.loglike()
-28238.336880999712
m.maximize_loglike()
Iteration 013 [Optimization terminated successfully]
Best LL = -25157.723517429302
value | initvalue | nullvalue | minimum | maximum | holdfast | note | best | |
---|---|---|---|---|---|---|---|---|
EmpNonRetail_HighInc | 1.363964 | 0.0 | 0.0 | -inf | inf | 0 | 1.363964 | |
EmpNonRetail_LowInc | -0.881402 | 0.0 | 0.0 | -inf | inf | 0 | -0.881402 | |
EmpRetail_HighInc | 0.000000 | 0.0 | 0.0 | 0.000 | 0.0 | 1 | 0.000000 | |
EmpRetail_LowInc | 0.000000 | 0.0 | 0.0 | 0.000 | 0.0 | 1 | 0.000000 | |
Theta | 0.749368 | 1.0 | 1.0 | 0.001 | 1.0 | 0 | 0.749368 | |
distance | -0.041820 | 0.0 | 0.0 | -inf | inf | 0 | -0.041820 | |
logsum | 1.020788 | 0.0 | 0.0 | -inf | inf | 0 | 1.020788 |
/home/runner/work/larch/larch/larch/larch/model/optimization.py:308: UserWarning: slsqp may not play nicely with unbounded parameters
if you get poor results, consider setting global bounds with model.set_cap()
warnings.warn( # infinite bounds # )
key | value | ||||||||||||||||
---|---|---|---|---|---|---|---|---|---|---|---|---|---|---|---|---|---|
x |
| ||||||||||||||||
loglike | -25157.723517429302 | ||||||||||||||||
d_loglike |
| ||||||||||||||||
nit | 13 | ||||||||||||||||
nfev | 32 | ||||||||||||||||
njev | 13 | ||||||||||||||||
status | 0 | ||||||||||||||||
message | 'Optimization terminated successfully' | ||||||||||||||||
success | True | ||||||||||||||||
elapsed_time | 0:00:01.530184 | ||||||||||||||||
method | 'slsqp' | ||||||||||||||||
n_cases | 7564 | ||||||||||||||||
iteration_number | 13 | ||||||||||||||||
logloss | 3.3259814274761106 |
m.calculate_parameter_covariance()
Model Visualization¶
For destination choice and similar type models, it might be beneficial to
review the observed and modeled choices, and the relative distribution of
these choices across different factors. For example, we would probably want
to see the distribution of travel distance. The Model
object includes
a built-in method to create this kind of visualization.
m.distribution_on_idca_variable('AUTO_DIST')
<AxesSubplot:xlabel='AUTO_DIST', ylabel='Relative Frequency'>
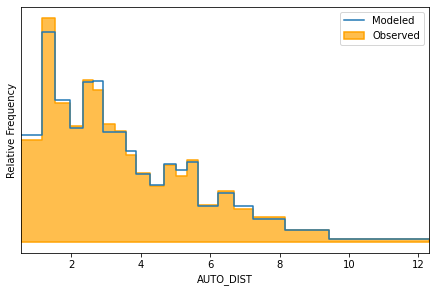
The distribution_on_idca_variable
has a variety of options,
for example to control the number and range of the histogram bins:
m.distribution_on_idca_variable('AUTO_DIST', bins=40, range=(0,10))
<AxesSubplot:xlabel='AUTO_DIST', ylabel='Relative Frequency'>
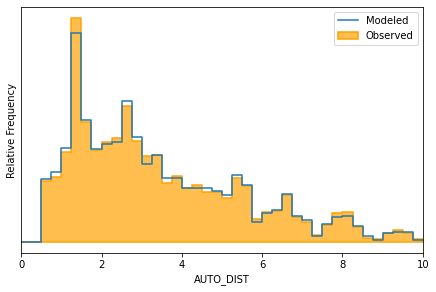
Alternatively, the histogram style can be swapped out for a smoothed kernel density function:
m.distribution_on_idca_variable(
'AUTO_DIST',
style='kde',
)
<AxesSubplot:xlabel='AUTO_DIST', ylabel='Relative Frequency'>
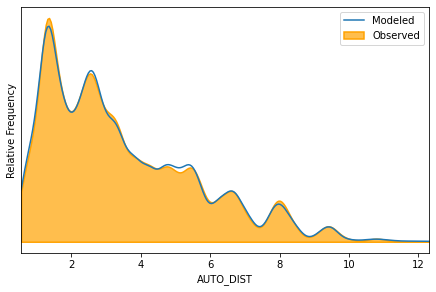
Subsets of the observations can be pulled out, to observe the
distribution conditional on other idco
factors, like income.
m.distribution_on_idca_variable(
'AUTO_DIST',
xlabel="Distance (miles)",
bins=26,
subselector='INCOME<10000',
range=(0,13),
header='Destination Distance, Very Low Income (<$10k) Households',
)
<AxesSubplot:xlabel='Distance (miles)', ylabel='Relative Frequency'>
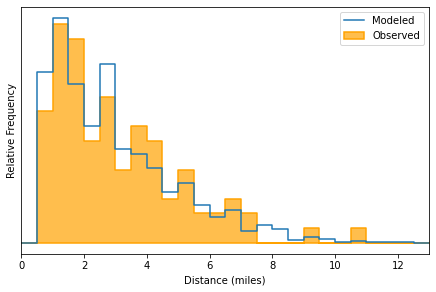
Save and Report Model¶
report = lx.Reporter(title=m.title)
report << '# Parameter Summary' << m.parameter_summary()
Parameter Summary
Value | Std Err | t Stat | Signif | Null Value | Constrained | |
---|---|---|---|---|---|---|
EmpNonRetail_HighInc | 1.36 | 0.256 | 5.32 | *** | 0.00 | |
EmpNonRetail_LowInc | -0.881 | 0.0791 | -11.14 | *** | 0.00 | |
EmpRetail_HighInc | 0.00 | NA | NA | 0.00 | fixed value | |
EmpRetail_LowInc | 0.00 | NA | NA | 0.00 | fixed value | |
Theta | 0.749 | 0.0152 | -16.45 | *** | 1.00 | |
distance | -0.0418 | 0.0107 | -3.90 | *** | 0.00 | |
logsum | 1.02 | 0.0317 | 32.16 | *** | 0.00 |
report << "# Estimation Statistics" << m.estimation_statistics()
report << "# Utility Functions" << m.utility_functions()
Utility Functions
+ P.logsum * X.logsum + P.distance * X.AUTO_DIST + P.Theta * log( + exp(P.EmpRetail_HighInc) * X('RETAIL_EMP * (INCOME>50000)') + exp(P.EmpNonRetail_HighInc) * X('NONRETAIL_EMP*(INCOME>50000)') + exp(P.EmpRetail_LowInc) * X('RETAIL_EMP*(INCOME<=50000)') + exp(P.EmpNonRetail_LowInc) * X('NONRETAIL_EMP*(INCOME<=50000)') ) |
The figures shown above can also be inserted directly into reports.
figure = m.distribution_on_idca_variable(
'AUTO_DIST',
xlabel="Distance (miles)",
style='kde',
header='Destination Distance',
)
report << "# Visualization"
report << figure
report.save(
'exampville_dest_choice.html',
overwrite=True,
metadata=m,
)
'exampville_dest_choice.html'